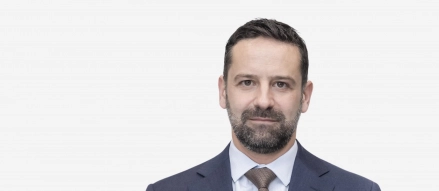
Will the emergence of big data analytics lead to a new wave of regulation in the financial sector?
Uncategorized
Will the emergence of big data analytics lead to a new wave of regulation in the financial sector?
Wed 18 Apr 2018
Big data analytics is one of the most discussed topics in the world of finance. Application of these methods has led to better assessment of credit quality, improved pricing of insurance contracts as well as automation of client interaction. Processes that were previously cumbersome, such as customer on-boarding, have been streamlined and their costs drastically cut. Institutions are even using Machine Learning (ML) to achieve greater regulatory compliance and detect fraud.
With all these benefits, however, comes a significant amount of new risks and threats to financial stability. Regulatory bodies such as the Financial Stability Board (FSB) and the European Banking Authority (EBA) have invested in the monitoring of the new advances. As a result, some concerns have been voiced about the lack of regulation around technologically-enabled innovation in the financial sector – or FinTech for short. This article considers a particular subset of FinTech – the application of ML and its impact on financial markets. We outline the benefits, risks and potential policy responses to the roll out of these tools.
In order to begin the discussion, it is important to define concepts. Below are the key definitions of terms used in this article. Figure 1 illustrates the intersection between them.
- Big data is defined as streams of data that are so large that they make traditional forms of analysis obsolete.
- Big data analytics refers to a collection of methods to make inference on big data.
- FinTech is innovation in the financial sector based on technological advancements. This can include big data methods as well.
- Machine Learning (ML) is a form of big data analytics which refers to the ability of machines to perform data tasks (e.g. group observations in clusters) without being specifically programmed.
Figure 1: Big Data, Machine Learning and FinTech
Adoption of big data methods such as ML by financial institutions are just beginning to bring efficiency savings for the sector. The application of these technologies is not limited to one area – they can be applied in fraud detection, risk management, customer service and regulatory compliance among others.
It is difficult to quantify the impact of ML on costs related to these activities, but the potential of big data is obvious. One example comes from fraud detection – as the Indian IT Services firm Wipro writes in a paper on ML and fraud detection: “The challenge with [traditional] approaches is that they rely very heavily on manual intervention which will lead to [multiple] limitations”.[1]
These limitations are a constraint to operating with a limited set of known parameters, inability to understand context-specific relationships between parameters and manual recalibration of existing models. ML can circumvent these issues by automating decisions based on insights from large data sets – something a human researcher would not be able to do. Wider application of ML could then cut labour costs when it comes to activities such as fraud detection.
Another useful application of ML is its potential to aid credit risk calculations for entities that do not have a prior credit history. The Final Report (15/03/2018) [2] on Big Data by the European Supervisory Authorities (ESAs) – consisting of the EBA, the European Insurance and Occupational Pensions Authority (EIOPA) and the European Securities and Markets Authority (ESMA) – pointed out that a significant number of survey respondents from the financial sector believed that this aspect of ML can enable fair and affordable access to financial services. Among the beneficiaries of these technologies are millennials, who may find it difficult to obtain credit due to a lack of credit history, or people who may get cheaper insurance premiums as a result of healthier lifestyles that their insurer can monitor.
Furthermore, respondents were optimistic about the potential of robo-advice which uses ML to help consumers compare options and choose the financial products most suited to their investment needs. Such services can contribute to more efficient financial markets as they not only enable consumers to choose the best product, but can also help investors compare prices of different financial instruments.
The benefits of ML are therefore undeniable and big regulatory bodies such as the EBA have officially recognised this. The challenges arising as a result of the new technologies, however, must also be factored in to truly understand the impact of innovation. The Financial Stability Board (FSB) identified several key risks in the application of Artificial Intelligence (AI) and ML in its reports from July and November last year. It highlighted that expansion of these particular aspects of FinTech may create new sources of volatility in financial markets. The lack of auditability of the algorithms used by financial institutions was identified as a key concern for future macro stability.
As Benoît Cœuré of the executive Board of the ECB said on 14 March 2018 during a roundtable organised by the German FinTech investor Finleap:
“The challenge for regulators is to balance the obvious benefits that fintech innovations may have for growth and welfare with their potential risks. After all, the history of financial innovation is littered with examples that led to early booms, growing unintended consequences, and eventual busts.”
These concerns appear to be shared by industry stakeholders as it became clear in the ESAs Final Report on Big Data. Among the main risks identified by respondents was the lack of transparency of algorithms used in products based on ML, as well as some price discrimination concerns in financial markets.
The EBA highlighted that the opacity of algorithms can prevent consumers from understanding certain products and creates asymmetry of information in favour of the supplier. In addition to this, the FSB reported in November that “The lack of interpretability or ‘auditability’ of AI and machine learning methods has the potential to contribute to macro-level risk if not appropriately supervised by microprudential supervisors.”
This is perhaps the most important issue arising from the increased use of ML. According to the FSB, most algorithms are trained using data from low-volatility periods, meaning that in times of economic stress, decisions based on big data models may have significant systemic implications.
The fact that these issues are recognised by international regulatory bodies is an important signal. Financial institutions that rely heavily on big data analytics and ML should be wary of regulatory developments as very soon, financial institutions may be subject to tightened standards around technology.
There is already some movement in this direction: the FSB reported that the International Organization of Securities Commissions and Senior Supervisors’ Group has recommended regulation on algorithmic trading to be considered. In addition, the EBA published on 15/03/2018 a Roadmap on Fintech, outlining its key priorities for monitoring in 2018-2019. One of those priorities is closer scrutiny of emerging trends and the analysis of prudential risks arising from FinTech, which is likely to include big data analytics.
Apart from the systemic implications of big data, regulators are concerned about consumer welfare in certain markets. ML has the potential to uncover relationships between observable characteristics of consumers and their likelihood to claim on a certain policy for example, or their propensity to buy a given financial product. Firms can then exploit this information in order to charge higher premiums for certain consumers or charge higher prices when they identify that a customer is time constrained.
Price discrimination of this type is not illegal and is part of the profit-maximising behaviour of firms. The availability of big data and ML, however, may make this much more prevalent, impacting the welfare of consumers, potentially leaving many people out of insurance markets.
The ESAs currently encourage institutions to promote non-discriminatory treatment of consumers. Concrete steps that firms can take are outlined in its Final Report on Big Data. Regulatory intervention, however, is considered to be premature. Nonetheless, just as some countries ban refusing health insurance on grounds of pre-existing conditions, very soon we may see regulations imposed on insurance pricing decisions made by machines.
The final consideration for the application of ML is data quality. Without good data, algorithm outputs can result in erroneous decisions and systemic risks. The FSB highlighted that as a lot of the data is supplied by third parties, there may be dependencies that fall outside the scope of current prudential regulation.
Respondents to ESAs’ survey shared this concern. They, however, also expressed optimism about new data standards such as GDPR in curbing those negative effects of big data analytics. Nonetheless, more heavy regulation may come about in the future as reliance on ML grows.
Big data analytics is changing the financial services environment fast. Costs are drastically cut and new services emerge as a result of technological innovation. But relying more on machines to make financial decisions is a risky process. If fed biased data, the most elegant of algorithms can produce misleading outputs. The current regulatory framework generally does not consider these impacts, but authorities are keeping abreast of new developments in the financial world. There is a growing sentiment towards more caution when it comes to applying new technologies in the financial sector.
Stakeholders in the financial markets should be aware of these sentiments as new types of regulation may be just around the corner. This should not be interpreted as bad news – it is the price to be paid for the many advantages that big data and ML bring to the world of finance.
[1] https://community.microstrategy.com/s/article/Comparative-Analysis-of-Machine-Learning-Techniques-for-Detecting-Insurance-Claims-Fraud2
[2] http://www.eba.europa.eu/-/esas-weigh-benefits-and-risks-of-big-data
Want to get notified when new blog posts are published?
Subscribe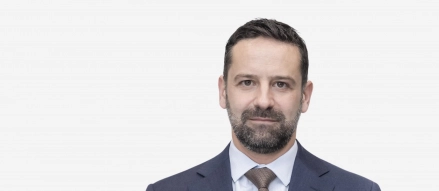